Evaluation of MODIS and Proba-V NDVI time series for mapping understory vegetation
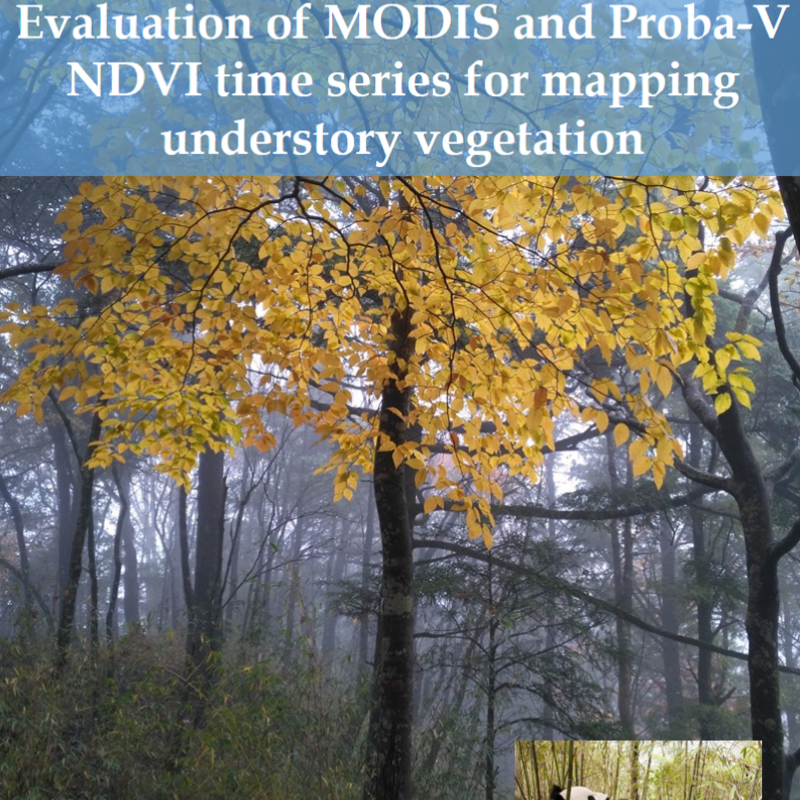
Field work can be organized and carried out in the Foping Giant Panda Nature Reserve in China, but not mandatory.
Understory vegetation is an important component in forest ecosystems not only because of its
contributions to forest structure, function and species composition, but also due to its essential role in supporting wildlife species such as the giant panda. Within the giant panda habitat, bamboo is found predominantly as understory species. In forested areas, bamboo is distributed in patches ranging in size from single plants to hundreds of meters across. However, the spatial distribution of bamboo seemingly does not follow any trends relative to overstory or abiotic factors. In addition, the understory bamboo can be evergreen or deciduous depend on the species, which make the prediction or classification of understory bamboo forests using traditional methods difficult and required a new approach for accurate classification. Previous studies demonstrated that time series of MODIS NDVI data has high capacity for characterization of plant phenology, and hence detection of evergreen understory bamboo. However, the spatial and temporal resolution of MODIS NDVI time series may be too coarse to capture the relatively small, isolated patches of understory bamboo forests, which are critical for the monitoring, protection and restoration of giant panda habitat.
In this study, we propose to use relatively high spatial resolution (i.e.,100 m) and very high temporal resolution (i.e., 5-day composites) Proba-V NDVI time series as well as its associated greenness metrics (e.g., maximum NDVI, mean NDVI, onset of greenness, and senescence of greenness), in combination with terrain factors (e.g., elevation, slope and aspect) to mapping understory bamboo forest in a giant panda habitat – Qinling Mountains. The Random Forests machine learning algorithm will be applied for this purpose. The bamboo classification results derived from Proba-V NDVI time series will be compared with the one derived from MODIS NDVI time series. In addition, the most important predictors and critical time period that contributed to the success of remote sensing of understory bamboo detection will be determined.