Machine Learning for Flood mapping and Flood early warning using high-resolution satellite rainfall estimates
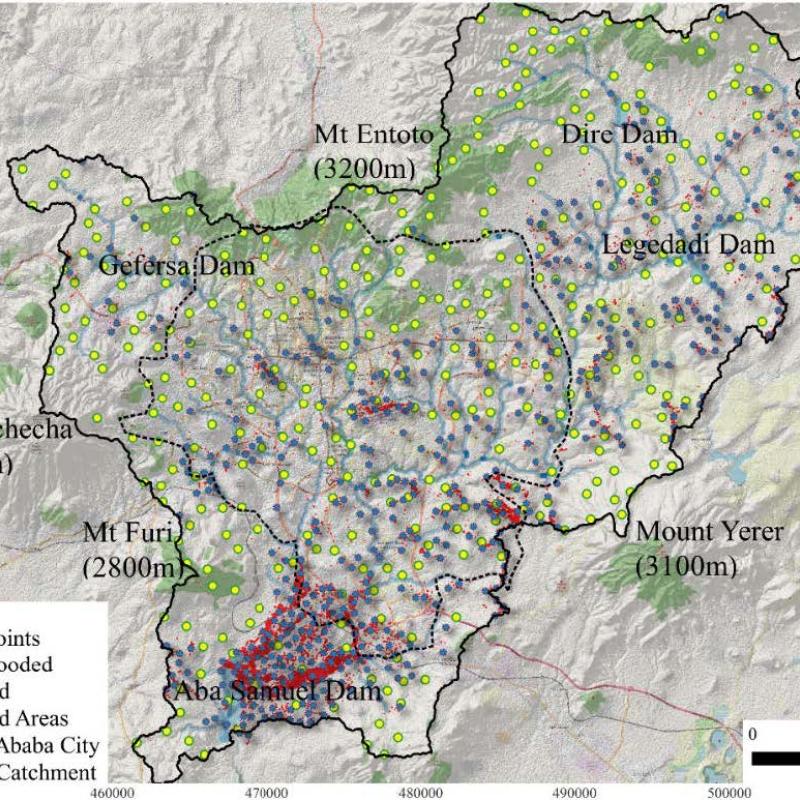
This study relies on Satellite based flood data indicating a recent pluvial flood events.
Accurate and reliable data is demanded in water, climate and environmental studies but such data cannot be guaranteed in many countries as observation networks have deteriorated. Although the use of satellite data has been advocated a lot to replace field observations, many products such as satellite rainfall estimated contain (large) systematic and random errors. This study evaluates machine learning for flood mapping and early warning using high-resolution SRE products. The aim is to develop a flood-early warning (forecasting) tool using satellite rainfall products among topographic and physiographic inputs.
Optimal use of multiple satellite products including urban pluvial flood maps and satellite rainfall as a reference is foreseen to train algorithms. The area of study can be an urbanized area in Africa, or any other area of interest. Below a map with registered Pluvial flood events (2017-2020) in Addis Abebe (Ethiopia).
The overall aim of this study is to develop a machine learning based flood early warning tool that employs various satellite data sources for model development, training and validation. This study (i) evaluates how and which satellite products can be used for flood prediction, ii) how well pluvial floodings can be simulated and iii) what forecasting window lengths (i.e. lead times) can be defined to still be effective. The Google Earth Engine environment will be used.