Crop and inter-cropping mapping in Ethiopia using UAV and satellite images
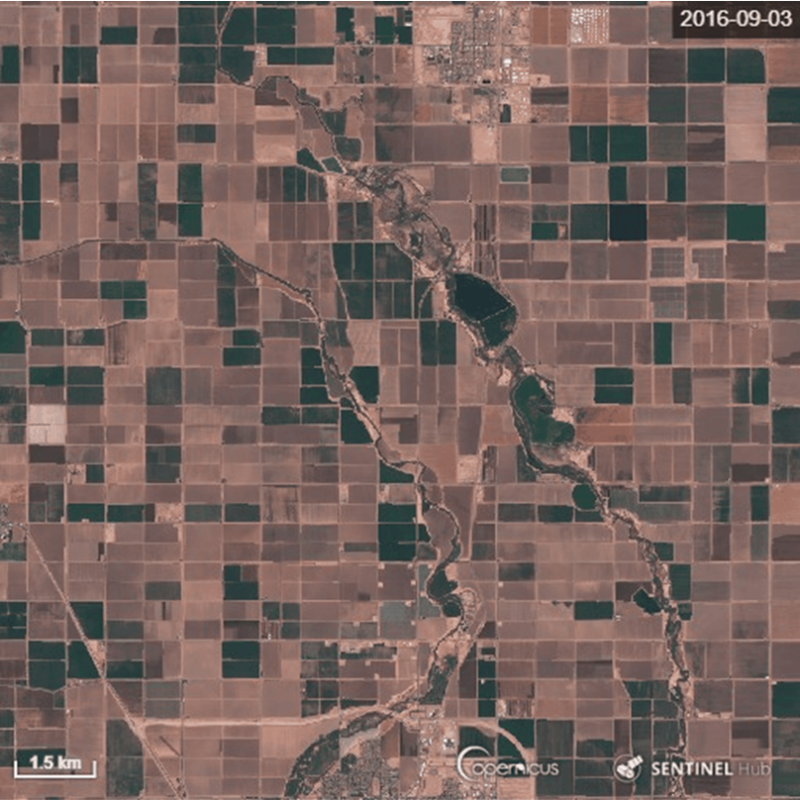
Prerequisite: programming skills are required to successfully implement this MSc research topic
Ethiopia’s diverse agricultural landscape is crucial to its economy and food security. However, accurate and scalable crop monitoring remains challenging due to the complexity of the agricultural landscape and limited access to high-resolution data. This MSc research focuses on mapping crop types and inter-cropping patterns in Ethiopia using a combination of Unmanned Aerial Vehicle (UAV) and satellite images, and advanced machine learning techniques.
Objectives:
- Implement advanced machine learning techniques to classify crops and inter-cropping systems and assess their spatial distribution and dynamics.
- Compare the effectiveness of UAV-derived imagery and satellite data for crop and inter-cropping mapping in different agroecological zones.
The study will use UAV images and complementary satellite data (e.g., Sentinel-2, Landsat 8) to map crop types and inter-cropping patterns in representative Ethiopian agricultural regions. Advanced machine learning algorithms will be implemented for crop and inter-cropping system mapping. This research will give you the opportunity to interact with a team of international collaborators from Ethiopia and France.
Mohammadi, S., Belgiu, M., Stein, A., 2024. A source-free unsupervised domain adaptation method for cross-regional and cross-time crop mapping from satellite image time series. Remote Sensing of Environment 314, 114385.
Van Tricht, K., Degerickx, J., Gilliams, S., Zanaga, D., Battude, M., Grosu, A., Brombacher, J., Lesiv, M., Bayas, J.C.L., Karanam, S., 2023. WorldCereal: a dynamic open-source system for global-scale, seasonal, and reproducible crop and irrigation mapping. Earth System Science Data 15, 5491-5515.