Predict diet diversity from space: socio-ecologically informed use of satellite imagery with machine learning
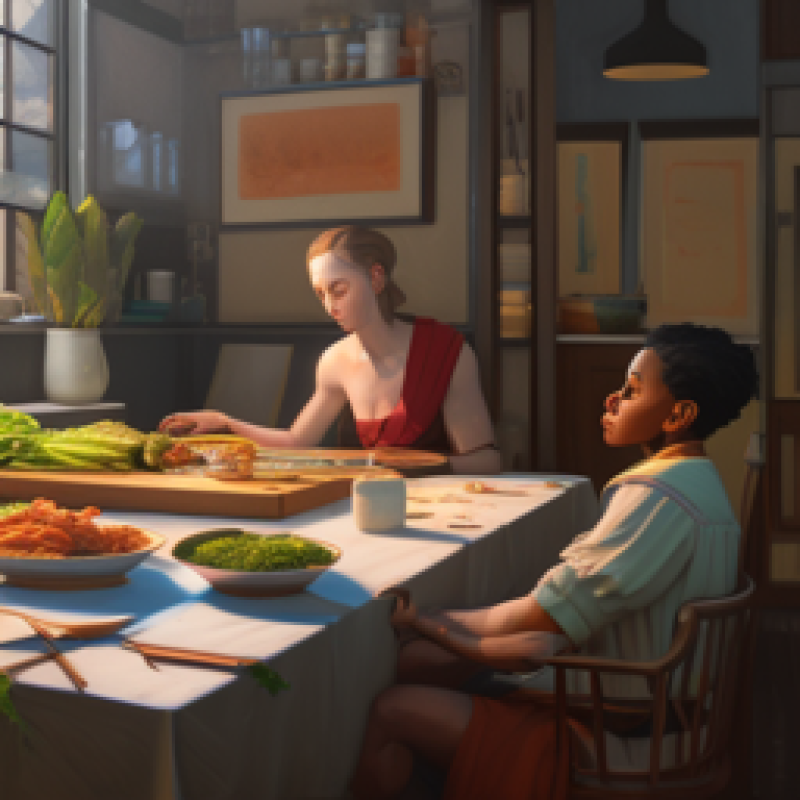
The topic is open for also suitable for GEM students in track 1 – GEM for Urban-Rural Interactions.
The topic is open for also suitable for GEM students in track 3 – GEM for Ecosystems & Natural Resources.
Suggested elective: Advanced Image Analysis
project image credit to Hotpot.ai using keywords: diet diversity
As an essential part of food security, diet diversity often requires frequent household food security surveys to monitor. Diet diversity is typically measured by the number and range of food groups consumed by households over a given time period, and is often considered an indicator of nutrition intake. To have a good estimation of the diet diversity of rural households, particularly in less-developed regions, asks for high-resolution and longitudinal household survey data with high financial and personal costs. In this topic, we will investigate the role that satellite data could have in monitoring and estimating diet diversity of rural households. To date, remote sensing data and methods have been used to predict indicators that traditionally require surveys to estimate, such as poverty. The use of remote sensing data for food security however is mostly on the availability dimension through crop extent and yield mapping, yet the role of RS in predicting the utilization dimension of food security has not been explored.
The MSc project answers the questions: (1) Can we use remote sensing data to predict rural household diet diversity, and to what degree can remote sensing data explain the variance in diet diversity among rural households in the same communities? (2) which variables are the most important ones when predicting diet diversity?
The MSc candidates will use existing geo-tagged household diet diversity data collected from countries in Africa (e.g., Zambia and Ghana), and process relevant RS data and socio-environmental data to derive relevant variables at multiple levels. For example, homestand at the individual household level, livestock, crop intensity and diversity at the landscape level, and food storage and road network at the village level. These variables from multiple levels will be fed into a machine-learning model, to predict the spatial distribution and variance of household diet diversity.
Jean, N., Burke, M., Xie, M., Davis, W. M., Lobell, D., & Ermon, S. (2016). Combining satellite imagery and machine learning to predict poverty. Science, 353(6301), 790–794. http://science.sciencemag.org.www2.lib.ku.edu/content/sci/353/6301/790.full.pdf
Watmough, G. R., Marcinko, C. L. J., Sullivan, C., Tschirhart, K., Mutuo, P. K., Palm, C. A., & Svenning, J.-C. (2019). Socioecologically informed use of remote sensing data to predict rural household poverty. Proceedings of the National Academy of Sciences, 116(4), 1213–1218. https://doi.org/10.1073/pnas.1812969116
Local policies related to diet diversity will be collected and analyzed.