A deep learning model for oil spill mapping
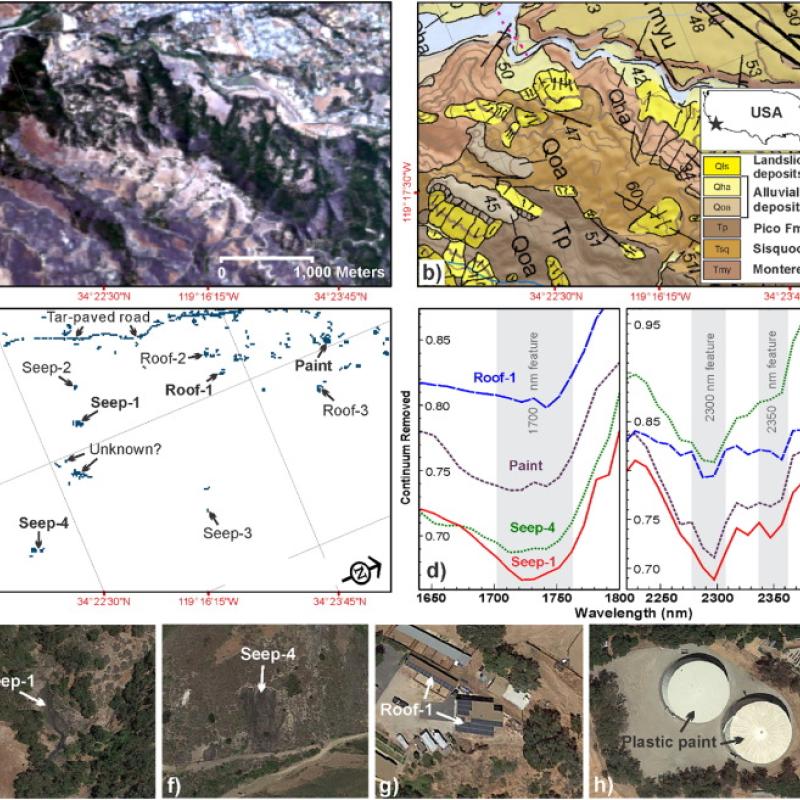
This MSc thesis topic is a collaboration with Dr. Saeid Asadzadeh, a member of the EnMap science team, at the German Research Centre for Geosciences (GFZ).
Global production, transportation, and consumption of oil can cause inevitable spills into the environment (typically from pipelines, oil wells, and storage facilities) contributing to surface and groundwater contamination. The hazardous and toxic contents of spilled petroleum can negatively impact flora and fauna, and human health, and accordingly, it should be immediately detected and remediated from the environment [1].
The advent of new satellite instruments with high spatial and spectral resolutions (e.g., WorldView-3 and EnMAP) has opened up new opportunities for terrestrial oil spill detection, mapping, and monitoring. The present challenge, however, is to adopt a robust processing algorithm capable of detecting the spectrally variable signal of oil with low reflectance properties and nonlinear spectral behavior in a heterogeneous background, which can also change over time due to degradation [2]. The supervised multi-class classifiers are not appropriate for this aim as they need all the classes (land cover types) in the scene to be known and incorporated in the training stage. However, one-class classification (OCC) techniques allow searching for the class of interest (target class) in a scene with the remaining classes being treated as unknowns (background clutter).
This topic aims to implement a workflow based on advanced OCC techniques. Particularly, we expect to develop a Deep One-Class Classification (DOCC) framework [3-4], which is found to be the state-of-the-art technique for OCC. The test data comprises WorldView-3 multispectral data over a contaminated site in the Qom region (Iran) and the Middle Magdalena Basin (Colombia) with known occurrences of natural oil seeps and anthropogenic contaminations. The study will also be extended to the EnMAP hyperspectral data planned to be acquired over the same sites as well as oil-polluted areas and oil fields in the Middle East.
[1] Merv Fingas, M., 2015, Handbook of Oil Spill Science and Technology, John Wiley & Sons. pp. 693.
[2] Asadzadeh, S., and C. R. Souza Filho, 2017, Spectral remote sensing for onshore seepage characterization: A critical overview: Earth-Science Reviews, v. 168, p. 48–72.
[3] P. Perera and V. M. Patel, "Learning Deep Features for One-Class Classification," in IEEE Transactions on Image Processing, vol. 28, no. 11, pp. 5450-5463, Nov. 2019.
[4] Lei, L., Wang, X., Zhong, Y., Zhao, H., Hu, X., & Luo, C. (2021). DOCC: Deep one-class crop classification via positive and unlabeled learning for multi-modal satellite imagery. International Journal of Applied Earth Observation and Geoinformation, 105, 102598.