Deep learning for assessing spatial-temporal variability of urban climate
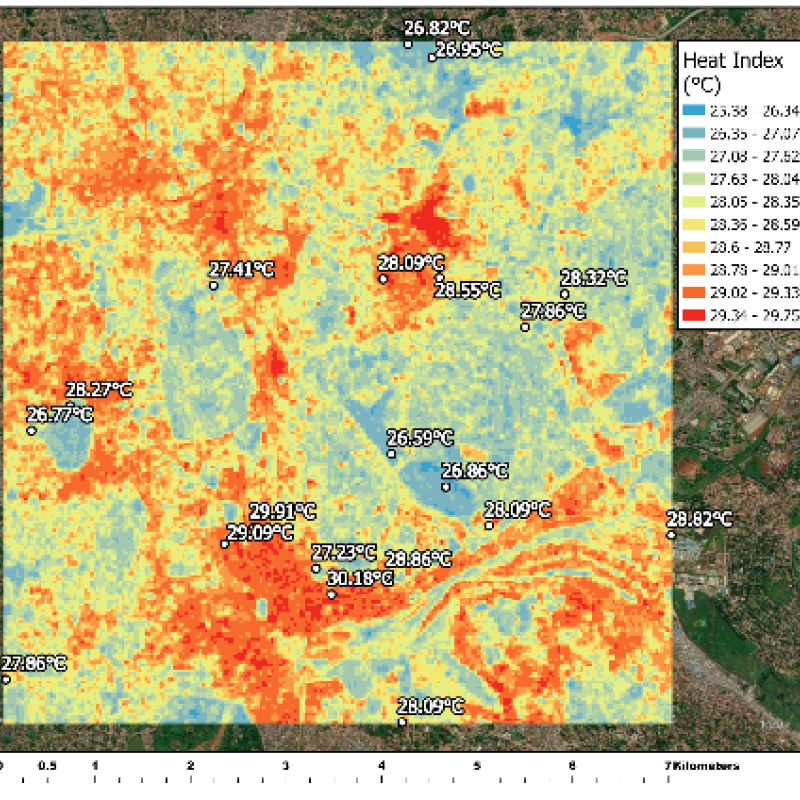
The climate in cities and metropolitan areas (i.e., urban climate) is often different from the climate in the surrounding rural areas due to the presence of buildings, pavement, and other urban infrastructure. Often, urban areas have higher temperatures compared to rural areas. Traditionally, urban climate research involves the use of monitoring stations located throughout urban areas, equipped with sensors for measuring various climate parameters (such as air temperature and relative humidity), and the application of geospatial analysis techniques to this measured point data. An analysis of suitable predictors for point data for a range of ambient conditions (e.g., temperature, humidity, thermal comfort), whilst accounting for temporal variability (diurnal and seasonal) would benefit the advancement of predictive modelling and mapping of climate in cities [1]. However, this subject remains yet to be fully resolved.
The advancement of Machine Learning (ML) for geospatial analysis is increasingly creating novel ways for combining interpolation with ML (see, e.g., in [2]). Particularly, Deep Learning (DL) techniques have shown to be a powerful tool in automatically extracting features from both spatial and temporal domains. However, nearly all available DL techniques that are developed for time series data prediction perform modeling at the locations of the measurement and not at any arbitrary spatial location. The DL-based prediction methodology presented in [3] seems to be one of the first attempts to perform prediction on a regular grid using sparsely spatially distributed ground measurements.
The aim of this MSc topic is to evaluate the performance of different predictor variables and predictive models for local-scale urban climate monitoring. The objectives of the project can be summarised as follows.
-Determine suitable predictors of local climate (based on spectral indices, land cover, and LST) across different time (diurnal and seasonal) scales
-Model the distribution of local urban climate on a regular grid using a deep learning-based method
-Evaluate the performance of the proposed method in view of other models using (spatial) cross-validation techniques
-Generate an analytical workflow that enables reproducibility for different datasets
Case study: You shall work with urban climate point data (air temperature, relative humidity, and heat index, to mention but a few) spanning 50 days, and possible complementary predictor data sets (e.g., spectral indices and land cover) derived from satellite imagery.
[1] Kabano P, Lindley S, Harris A. Evidence of urban heat island impacts on the vegetation growing season length in a tropical city. Landscape and urban planning. 2021 Feb 1;206:103989.
[2] Mallik S, Bhowmik T, Mishra U, Paul N. Mapping and prediction of soil organic carbon by an advanced geostatistical technique using remote sensing and terrain data. Geocarto International. 2022 Apr 18;37(8):2198-214.
[3] Amato F, Guignard F, Robert S, Kanevski M. A novel framework for spatio-temporal prediction of environmental data using deep learning. Scientific reports. 2020 Dec 17;10(1):22243.