Slum mapping using citizen science, deep learning and transfer learning methods
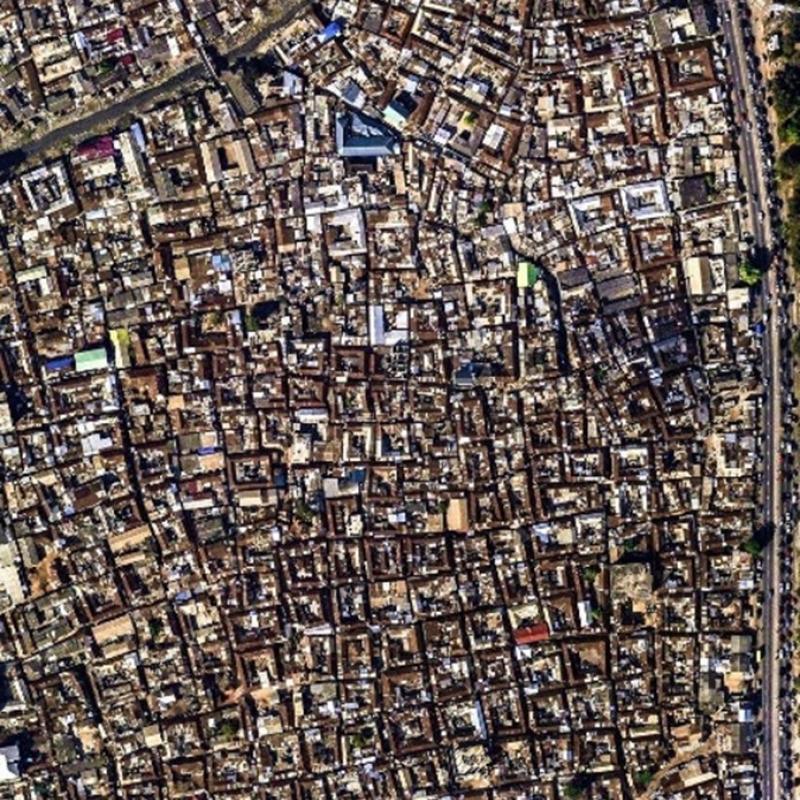
Suggested elective courses: Advanced image analysis
We are looking for talented MSc students who are willing to work in a multidisciplinary team.
Joint project with Anne Dijkstra from BMS (UT).
Mapping slums in different urban environments across the Globe is extremely important for locating and prioritizing hotspots of urban poor for urgent climate action to combat the impact of climate change. Earth Observation images together with advanced machine learning methods are key solutions used for this task. Yet, these solutions are mostly siloed, and they often lack transferability and scalability and fail to include the opinions of different interest groups. They are mostly top-down, with very little attention given to ground information and interaction with urban communities and stakeholders. Existing top-down approaches should be complemented with bottom-up approaches to produce routinely updated, accurate, and timely slum maps.
The overall goal of this MSc research is to use Earth Observation images and information on the slum locations and their socio-economic characteristics collected with the help of local communities to train deep learning algorithms (e.g. Transformers). Transfer learning methods such as few-shot learning (but not limited to) will also be investigated to increase the transferability of the trained model across different urban areas. This MSc research will give the students an unique opportunity to collaborate closely with a multidisciplinary team that is working on using Citizen Science, Earth Observation and Artificial Intelligence (AI) for mapping climate vulnerabilities of slums.
Zhuang, F., Qi, Z., Duan, K., Xi, D., Zhu, Y., Zhu, H., Xiong, H., & He, Q. (2020). A comprehensive survey on transfer learning. Proceedings of the IEEE, 109, 43-76