Few-shot learning for resilience in slums
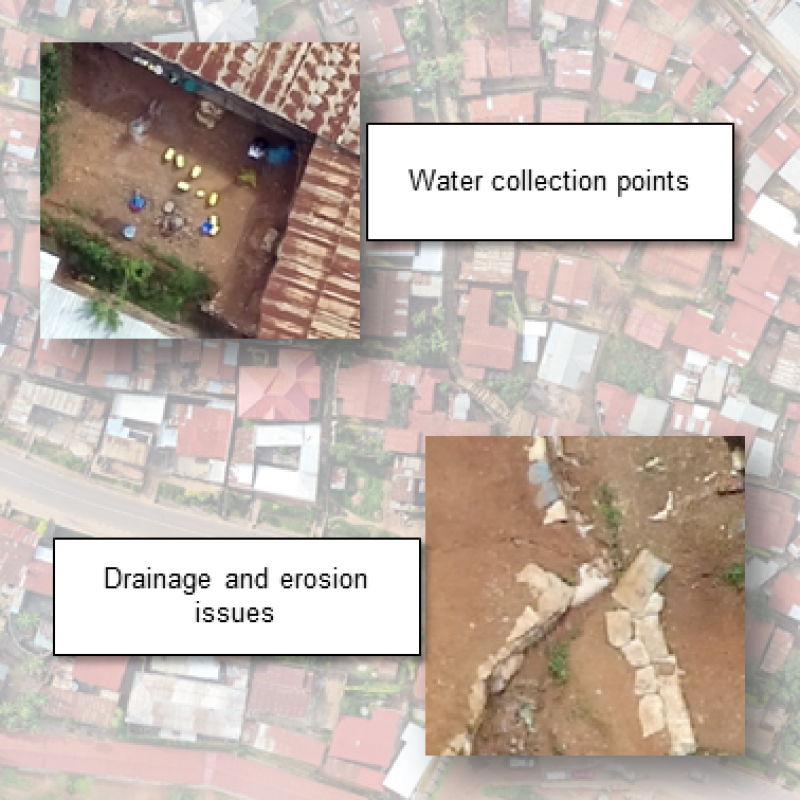
Suggested elective courses: Advanced image analysis
Programming skills required
UN-Habitat estimates that over one billion people around the world live in slums, which are often located in hazard-prone areas. Improving the living conditions in slums and is a priority for sustainable development, yet it is challenging as there is usually no information on what is going on inside the slum. Drones can be used to collect imagery and map the houses and basic infrastructure within slums. Indeed, as the images have a resolution of mere centimeters it is possible to see many more objects which can give important information on the vulnerability of slum residents. For example, sand-bags can indicate local flooding problems, and accumulations of solid waste can indicate health hazard.
Capturing such objects with deep-learning is challenging because there are no training datasets to recognize these objects. The link between objects visible in drone imagery and what that says about the social or physical vulnerability of the inhabitants of the slum depends strongly on the local context. N-shot or few-shot learning mechanisms can therefore be used to make deep learning more inclusive and more useful for local stakeholders.
To develop few-shot learning pipelines to identify novel objects in drone imagery of slums.
Shaban , A. Bansal, S, Liu, Z., Essa, I, & Boots, B. (2017). One-shot learning for semantic segmentation. arXiv:1709.03410
Wang, Y., Tian, X., & Zhong, G. (2022). FFNet: Feature Fusion Network for Few-shot Semantic Segmentaiton. Cognitive Computation 14, 875-886. https://doi.org/10.1007/s12559-021-09990-y