EO-Based Machine Learning for Essential Water Variables
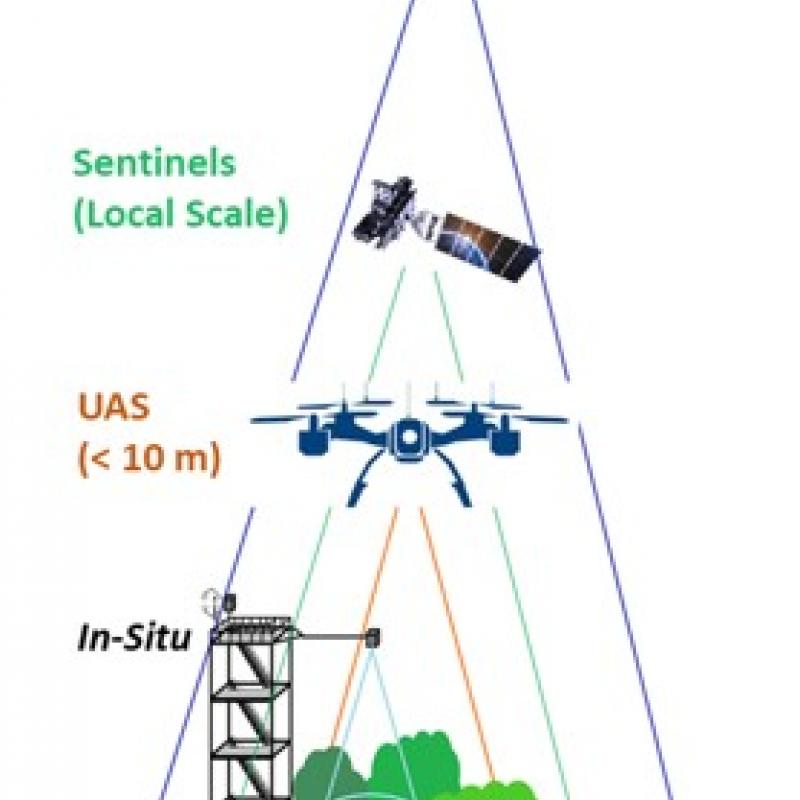
The past decades have seen rapid advancements in space-based monitoring of essential water cycle variables, providing products related to precipitation, evapotranspiration, and soil moisture, often at tens of kilometre scales. Whilst these data effectively characterize water cycle variability at regional to global scales, they are less suitable for sustainable management of local water resources, which needs detailed information to represent the spatial heterogeneity of soil and vegetation. The following questions are critical to effectively exploit information from remotely sensed and in situ Earth observations (EOs): How to downscale the global water cycle products to the local scale using multiple sources and scales of EO data? How to explore and apply the downscaled information at the management level for a better understanding of soil-water-vegetation-energy processes? How can such fine-scale information be used to improve the management of soil and water resources?
A recent example is the 2018 summer drought, which posed challenges for water availability and management in vast regions in Europe, including some ill-prepared to cope with water scarcity. Climate change presents additional challenges regarding the preparedness and adaptation to future extremes, because e.g. similar or worse future events to that in 2018 may be expected more frequently.
This MSc topic is aiming to close the gaps between satellite water cycle products and local information necessary for sustainable management of water resources.
The MSc research will aim to address the abovementioned scientific question by combining medium-resolution (10 m–1 km) Copernicus satellite data with high-resolution (cm) unmanned aerial system (UAS) data, in situ observations, analytical- and physical-based models, as well as big-data analytics with machine learning algorithms.
Su, Z.; Zeng, Y.; Romano, N.; Manfreda, S.; Francés, F.; Ben Dor, E.; Szabó, B.; Vico, G.; Nasta, P.; Zhuang, R.; Francos, N.; Mészáros, J.; Dal Sasso, S.F.; Bassiouni, M.; Zhang, L.; Rwasoka, D.T.; Retsios, B.; Yu, L.; Blatchford, M.L.; Mannaerts, C. An Integrative Information Aqueduct to Close the Gaps between Satellite Observation of Water Cycle and Local Sustainable Management of Water Resources. Water 2020, 12, 1495. https://doi.org/10.3390/w12051495
A mix of data sources has to be used of various spatial and spectral resolution in which SIS skills are needed.