Predicting Crop Yield with Hyperspectral versus Multispectral Broadband Image Data
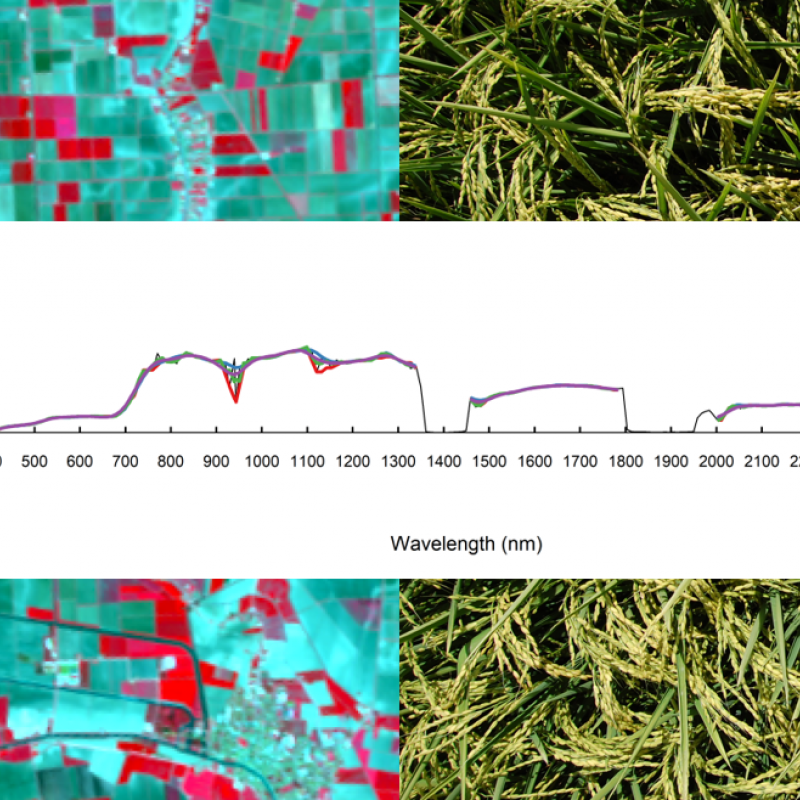
Statistics, agronomy and programming background/experience recommended.
Farmers, agronomists, private enterprise and policy-makers need to know field conditions with sufficient early-warning. Such information can, for example, help them target fertilizer, irrigation and pesticides. Earth observation image data is increasingly used for agricultural monitoring and modelling, because satellite images capture conditions on the ground remotely, consistently, and frequently over large areas. Multispectral broadband vegetation indices, such as the Normalized Difference Vegetation Index (NDVI) have been widely used to predict crop yields at the main stages of crop development, but fail to achieve the accuracies needed on agricultural DSSs. Hyperspectral Earth observation is going through a renaissance with the recent launches of Prisma and DESIS and anticpated launches of EnMAP, CHIME, and NASA Surface Biology and Geology (formerly HyspIRI). The unpresented spectral detail afforded by such platforms will undoubtably improve crop yield prediction, but by how much is not well known.
The purpose of the study is to develop predictive maps of crop yield for four world staples (corn, rice, soybean and wheat). Crop yield data for these crops was collected from several fields in the Province of Ferrara, Italy in 2020. The maps will be derived from two hyperspectral image sources (Prisma and DESIS) and two multispectral broadband image sources (Sentinel-2 and Landsat-8). The images are available at the main stages of crop development (emergence, peak productivity and senescence). Any number of data-driven (support vector machine, Random Forest, boosted regression) or light-use efficiency (SCOPE) modelling methods can be employed to relate spectral reflectance at the main stages of crop development to crop yield. We expect spectral performance will be highest during peak productivity, which is some months before harvest. Such information could help improve agricultural decision support systems (DSSs), such as the MARS Crop Yield Forecasting System.
Marshall, M. and Thenkabail, T.S. 2015. Advantage of hyperspectral EO-1 Hyperion over multispectral IKONOS, GeoEye-1, WorldView-2, Landsat ETM+, and MODIS vegetation indices in crop biomass estimation. ISPRS Journal of Photogrammetry and Remote Sensing, 108: 205-218.